Wind for Power Generation Wang Ping

Dynamic power flow algorithm considering frequency
With the primary frequency regulation characteristics of wind turbines, a simplified DPF algorithm is proposed in this study for power systems integrating wind power generation. The IEEE 30-bus system is modified to

Flexible Voltage Support Control with Imbalance Mitigation
Yuewu Wang†, Ping Yang*, Guangzhou, China **National-Local Joint Engineering Laboratory for Wind Power Control and Integration Technology, South China University of Technology,

Deep belief network based deterministic and probabilistic wind
The results demonstrate that the proposed ELM-based probabilistic forecasting method for wind power generation with a high potential for practical applications in power systems is effective

Simulation of a novel wind–wave hybrid power generation system
DOI: 10.1016/J.ENERGY.2021.121833 Corpus ID: 238689960; Simulation of a novel wind–wave hybrid power generation system with hydraulic transmission @article{Wang2022SimulationOA,

[PDF] Accelerating the energy transition towards photovoltaic and
The results show that if emissions peak in 2025, the carbon neutrality goal calls for a 45–62% electrification rate, 47–78% renewable energy in primary energy supply, 5.2–7.9

IET Renewable Power Generation
Recently, the techniques of levelling wind power by using KE storage within VSWTs have also drawn wide attention [20, 22, 26, 36, 52-54, 64-69]. Howlader et al. [20, 26] proposed the wind power levelling strategy by

Towards machine learning applications for structural load and
The mean wind speed at turbine locations is then extracted, allowing power generation to be estimated using manufacturer-provided power curves. However, this approach has limitations

Wind Power Forecasting with Deep Learning Networks: Time
long-term (24–72-h ahead) prediction of wind power with an MAPE of less than 10% by using the Temporal Convolutional Network (TCN) algorithm of DLNs. In our experiment, we performed

Design of backstepping power control for grid‐side
The entire framework of a wind power generation system with the type of DFIG for the grid connection via VSC-HVDC is depicted in Fig. 1, which consists of a wind farm, a wind-farm-side filter, a WFVSC, a high-voltage dc
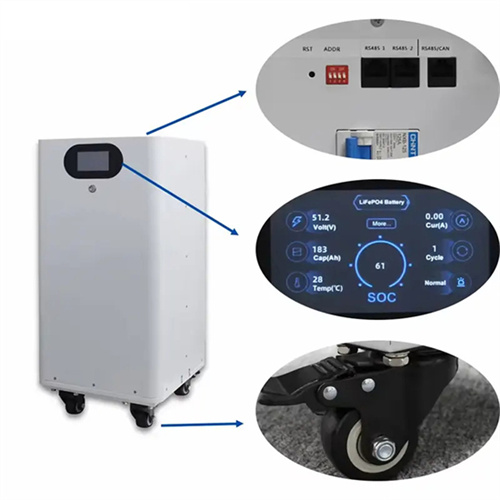
Deep belief network based k-means cluster approach for short-term wind
DOI: 10.1016/J.ENERGY.2018.09.118 Corpus ID: 117182219; Deep belief network based k-means cluster approach for short-term wind power forecasting @article{Wang2018DeepBN,

Utilisation of kinetic energy from wind turbine for grid connections:
1.1 Overview of WT level control. The turbine level control methods can be further divided into two categories, as shown in Fig. 1.One coordinates auxiliary devices such

Dynamic power flow algorithm considering frequency
Ruohan Wang. Key Laboratory of Power System Intelligent Dispatch and Control of the Ministry of Education, Shandong University, Jinan, 250061 People''s Republic of China. a simplified DPF algorithm is proposed

A Review of Modern Wind Power Generation
Wind power prediction involves applying state-of-the-art algorithms to the field of wind power generation so that wind power generation can be better connected to the electricity grid, and key technologies have

6 FAQs about [Wind for Power Generation Wang Ping]
Can machine learning predict wind power?
In recent years, machine learning models have been widely used in WPF as they can reveal complex nonlinear relationships between in historical data and other explanatory variables. Wang et al propose an adaptive robust multi-core regression model based on Bayesian to yield the deterministic and probabilistic prediction of wind power.
How accurate are wind power prediction models based on deep learning?
The recommended hybrid CNN model showed unsatisfactory accuracy in short-term wind power predictions with several deep learning frameworks, with an RMSE and MAE of 27.1% and a MAPE of 16.87%, respectively. The accuracy of the prediction models should be improved in further studies. 4.5. Statistical-Analysis-Based Approaches
Why do wind turbines convert PV nodes to PQ nodes?
After the normal generators at buses 2, 5 and 8 are replaced by wind turbines, these buses are transformed from PV nodes to PQ nodes. The phenomenon probably results from the lack of reactive power at these buses since wind turbines absorb reactive power from the system.
What are hybrid wind power prediction methods based on deep learning?
Table 5 summarizes the hybrid wind power prediction methods based on deep learning in the reviewed works. Table 5. Summary of Deep-learning (DL)-based approaches for wind power forecasting. Hybrid predictive models combine two or three deep learning techniques or include optimization algorithms.
How to predict wind power output?
The prediction of wind power output is part of the basic work of power grid dispatching and energy distribution. At present, the output power prediction is mainly obtained by fitting and regressing the historical data. The medium- and long-term power prediction results exhibit large deviations due to the uncertainty of wind power generation.
How can machine learning improve wind speed prediction?
Machine-learning-based methods are emerging prediction methods that can establish accurate models to describe nonlinear relationships, predict the essence of wind energy, and improve prediction accuracy. The use of machine learning techniques with optimization algorithms is very effective for day-ahead wind speed prediction.
Related Contents
- Kenya hybrid wind solar power generation
- Vietnam solar and wind hybrid power generation
- Wind power generation to solar power generation
- Xichang wind power generation
- China s photovoltaic power generation and wind power
- Latest news on wind power generation period
- Ranking of wind power generation in various countries
- Is wind power generation hereditary
- Wind power generation 690 AC
- Jiuding Tiandi Wind Power Generation Company
- Wind blade power generation under construction
- Theoretical power generation of wind farm